In a world where artificial intelligence propels innovation at an unprecedented pace, the ethical implications surrounding large language models (LLMs) have emerged as paramount considerations. Navigating the complex landscape of these powerful tools demands a profound understanding of the ethical conundrums they present. Delving into the heart of AI ethics, we embark on a journey to unravel the entwined threads of potential and pitfalls in harnessing LLMs. As we tread this intricate path, it becomes increasingly evident that steering with moral compasses is not merely advisable but imperative in shaping a responsible AI ecosystem.
Table of Contents
- 1 Navigating Ethics in Large Language Models: A Comprehensive Guide
- 2 Ethical Implications of Large Language Models
- 3 Strategies for Ethical Development of Large Language Models
- 4 Societal Impact of Large Language Models
- 5 Best Practices for Ethical Deployment of Large Language Models
- 6 Wrapping Up
- 7 Frequently Asked Questions About Ethical Considerations in Large Language Models:
- 7.1 Q: How does bias manifest in large language models?
- 7.2 Q: What are some common privacy concerns related to large language models?
- 7.3 Q: How can organizations combat misinformation spread by large language models?
- 7.4 Q: Why is it important to involve ethicists in the development process of LLMs?
- 7.5 Q: How can feedback loops enhance the ethical deployment of large language models?
The sprawling capabilities of large language models evoke awe and intrigue, yet beneath their seemingly limitless aptitude lies a realm fraught with ethical dilemmas. From bias lurking within algorithmic structures to the perpetuation of misinformation under their expansive wings, the implications are multifaceted and far-reaching. Striking a delicate balance between technological advancement and ethical integrity stands as an arduous task necessitating nuanced perspectives and proactive measures.
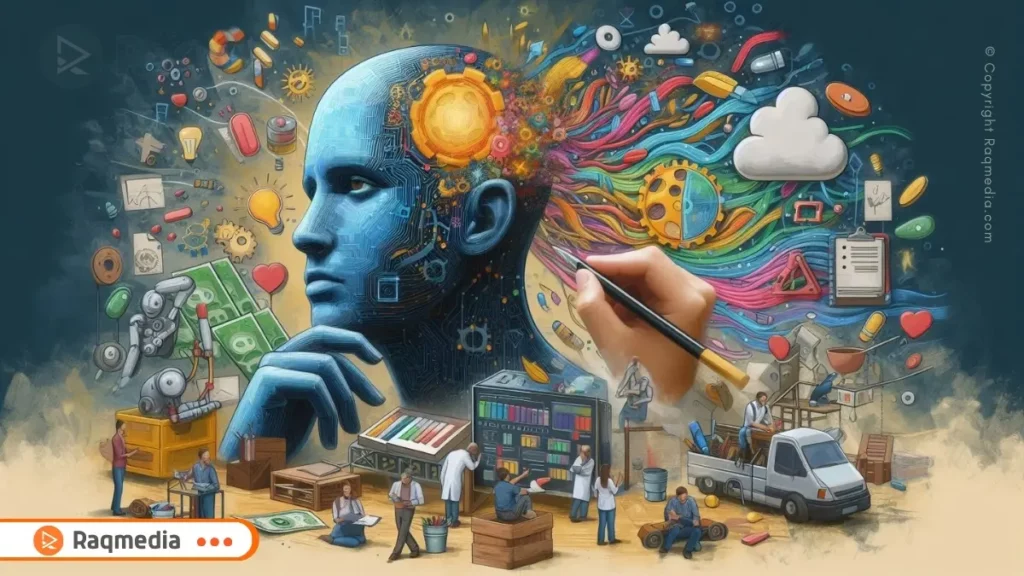
As we delve deeper into this comprehensive guide on navigating ethics in LLMs, we embark on a transformative exploration that transcends conventional boundaries to forge a path illuminated by ethical scrutiny and innovative solutions.
Ethical Implications of Large Language Models
In the realm of large language models (LLMs), an ethical concern that looms large is the impact of bias. These models, powered by vast datasets, can inadvertently perpetuate or even amplify societal biases present in the data they are fed. Take, for instance, a language model trained on historical texts where gender roles were skewed – this could lead to biased outputs favoring stereotypical gender assumptions in current text generation. The consequences of such biases can be profound, influencing decisions made based on LLM-generated content and perpetuating inequality.
Regularly reassess your ethical framework in light of new developments or challenges within the field.
Moreover, the rise of fake news and misinformation poses significant challenges when it comes to deploying large language models responsibly. Misinformation spread through AI-generated content can have far-reaching effects on public discourse, political stability, and individual beliefs. To tackle this issue effectively, developers must implement robust mechanisms for fact-checking and vetting information generated by these models before dissemination to prevent malicious actors from exploiting their capabilities for spreading false narratives.
Privacy concerns also come to the fore when discussing the development and deployment of large language models. With access to massive amounts of user data for training purposes, there is a risk that sensitive information may be inadvertently exposed or misused if adequate safeguards are not in place. Ensuring data security and privacy protections throughout the lifecycle of LLMs is crucial to maintain user trust and comply with regulatory frameworks designed to safeguard personal information from exploitation or unauthorized access.
Strategies for Ethical Development of Large Language Models
In the realm of large language models (LLMs), fostering ethical development practices is paramount to building systems that serve society equitably. Transparency measures play a crucial role in this endeavor, ensuring that users understand how these models operate and the potential biases they may exhibit. For instance, OpenAI’s GPT-3 model gained attention for its impressive language generation capabilities; however, concerns arose regarding unseen biases within its data sources. By enhancing transparency through detailed documentation on data sources and model training processes, developers can instill trust in their LLMs.
Moreover, inclusivity and fairness are essential principles when considering the data used to train LLMs. A notable example is Google's BERT model, which significantly improved natural language understanding but faced criticism for reinforcing gender stereotypes due to biased training data. To address this, developers should constantly evaluate and refine datasets to ensure representative and non-discriminatory content. By actively seeking diverse perspectives in dataset curation, teams can mitigate bias and promote inclusivity in their LLMs' outputs.
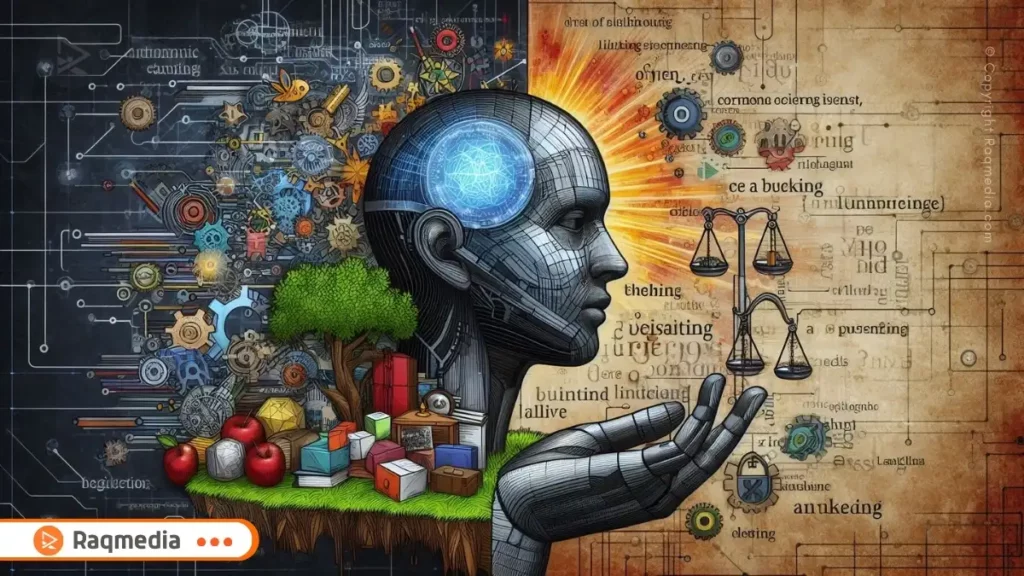
Collaboration with ethicists and stakeholders forms another vital strategy in the ethical development of large language models. Engaging experts from various backgrounds helps evaluate ethical risks comprehensively and anticipate unintended consequences. For instance, partnering with linguists, psychologists, ethicists, and community representatives can provide multidimensional insights into the impacts of LLM deployment across different societal contexts. This proactive approach not only enhances the ethical robustness of LLMs but also demonstrates a commitment to addressing complex ethical dilemmas that arise in AI technologies.
Societal Impact of Large Language Models
Large Language Models (LLMs) wield significant influence over societal norms and behaviors due to their widespread use in various applications. These models, if not carefully monitored, can perpetuate or amplify biases present in the data they are trained on. For instance, if a language model is predominantly fed biased text data that reflects discriminatory attitudes, it can inadvertently reinforce and normalize these biases when generating responses or content. This amplification effect highlights the critical need for ethical oversight in LLM development to prevent harmful societal repercussions.
Foster a culture that prioritizes ethical considerations in all stages of developing and deploying large language models.
The potential consequences of deploying biased language models at scale are far-reaching. They can exacerbate existing inequalities and discrimination by perpetuating stereotypes or marginalizing certain groups. Imagine a chatbot used in customer service that consistently provides unfavorable responses based on gender or race due to biased training data—such scenarios could severely impact user experiences and trust. To mitigate these negative societal impacts, developers must proactively address bias during the design phase and continuously monitor and adjust algorithms to ensure fairness across diverse user groups.
Responsible usage guidelines play a pivotal role in mitigating the negative societal effects of large language models. By establishing clear ethical standards and practices for developing and deploying LLMs, organizations can uphold principles of inclusivity, fairness, and transparency. For example, implementing diversity initiatives within training datasets can help reduce bias, while incorporating explainability features into models allows users to understand how decisions are made. Through collaborative efforts among developers, ethicists, policymakers, and communities, it becomes possible to navigate the complex landscape of ethical considerations in large language model applications sustainably.
Best Practices for Ethical Deployment of Large Language Models
In the realm of large language models (LLMs), establishing clear guidelines for ethical use is paramount. Companies and developers must outline explicit protocols on how these models should be deployed, ensuring that they align with ethical standards. For instance, setting boundaries on the types of content that could be generated by an LLM to prevent harmful outputs can help mitigate potential negative impacts. By creating a well-defined ethical framework, organizations can navigate the complexities of deploying these powerful tools responsibly.
Moreover, incorporating feedback loops into the deployment process can significantly enhance ethical practices surrounding LLMs. This mechanism allows for continuous monitoring and evaluation of how these models interact with users and data sources. For example, implementing real-time monitoring systems that flag any instances of bias or misinformation produced by an LLM enables prompt corrective actions to be taken. By iteratively refining their ethical deployment strategies based on feedback and performance metrics, organizations can adapt to evolving challenges effectively.
Stay engaged with industry-wide discussions on ethics in AI to remain informed about evolving best practices.
Engaging with diverse communities serves as another vital component in ensuring the ethical deployment of large language models. By soliciting input from various user groups with unique perspectives and concerns, organizations can gain valuable insights into how LLMs may impact different populations differently. For instance, hosting focus groups or conducting surveys among marginalized communities can shed light on potential biases or unintended consequences that may arise from deploying these models. Through inclusive dialogue and collaboration with stakeholders from diverse backgrounds, companies can tailor their ethical deployment practices to address a broader spectrum of societal needs and values.
Wrapping Up
In conclusion, navigating ethics in large language models (LLMs) requires a multifaceted approach that encompasses transparency, inclusivity, collaboration, and continuous improvement. By following best practices for ethical deployment of LLMs, organizations can mitigate the risks associated with bias, misinformation, and privacy concerns while maximizing the potential benefits of these powerful tools. Establishing clear guidelines for ethical use and monitoring compliance is essential to build trust with users and stakeholders. Incorporating feedback loops allows for ongoing assessment and refinement of ethical practices based on real-world outcomes.
Furthermore, engaging with diverse communities and incorporating their perspectives into the development and deployment of LLMs is crucial for ensuring inclusivity and fairness. By collaborating with ethicists and stakeholders from various backgrounds, organizations can evaluate ethical risks comprehensively and implement strategies to address them proactively. Responsible usage guidelines play a key role in minimizing negative societal impacts of biased language models by guiding developers, researchers, and end-users towards ethical decision-making.
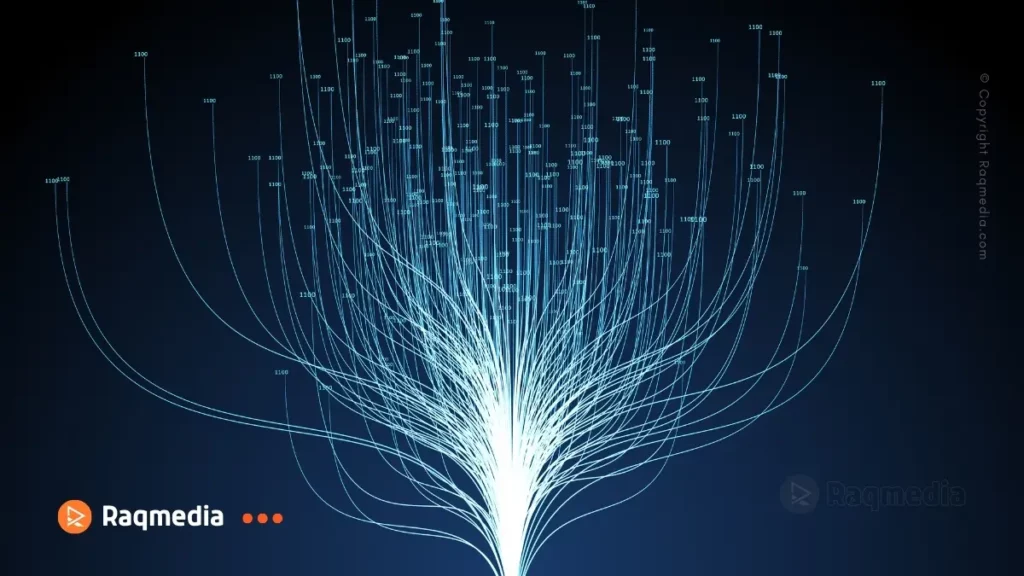
As the tech landscape evolves rapidly, staying attuned to emerging ethical considerations in large language models is paramount. Striking a balance between innovation and ethics will not only foster credibility but also contribute to the responsible advancement of AI technologies. Embracing an ethical framework in harnessing the potential of LLMs paves the way for a more sustainable future where technology serves society ethically and responsibly.
By embodying a commitment to transparency, inclusivity, collaboration, continuous improvement, and responsible usage guidelines in every aspect of working with large language models, organizations can navigate the complex terrain of ethics successfully while unlocking the vast capabilities these models offer for positive impact across industries.
Frequently Asked Questions About Ethical Considerations in Large Language Models:
Q: How does bias manifest in large language models?
A: Bias can be introduced through skewed datasets used in training LLMs, leading to discriminatory outcomes in generated content.
A: Privacy issues may arise from data handling practices during training or unintended disclosures of sensitive information by deployed LLMs.
Q: How can organizations combat misinformation spread by large language models?
A: Implementing fact-checking mechanisms within LLMs or partnering with experts to verify content output can help combat misinformation effectively.
Q: Why is it important to involve ethicists in the development process of LLMs?
A: Ethicists bring valuable insights into identifying and mitigating potential ethical risks posed by LLMs early on in their development cycle.
Q: How can feedback loops enhance the ethical deployment of large language models?
A: Feedback loops enable organizations to gather insights from users' experiences with LLMs, allowing for adjustments that improve ethical practices over time.