In the vast realm of artificial intelligence, two compelling approaches reign supreme: generative AI and predictive AI. Welcome to the captivating world of ‘AI Showdown: Generative vs Predictive – What's Your Style?' where we embark on a riveting journey through the contrasting landscapes of creativity and foresight. For tech enthusiasts, AI professionals, data scientists, and curious students alike, grasping the nuances between these distinctive AI styles is not merely an intellectual exercise – it's a gateway to unlocking unprecedented possibilities in the digital age.
Table of Contents
- 1 Generative AI vs Predictive AI – What's The Difference?
- 2 Generative AI: Fostering Creativity Through Data Transformation
- 3 Predictive AI: Shaping Tomorrow with Data Insights
- 4 Performance Comparison: Generative vs. Predictive AI
- 5 User Perspective: Choosing Your Style
- 6 Future Trends in AI Development
- 7 Generative AI's Creative Prowess In Question
- 8 Final Tips
- 9 Choosing Your AI Style
- 10 FAQs
- 10.1 1. Q: What are some key differences between generative and predictive AI?
- 10.2 2. Q: How do ethical concerns come into play when discussing generative vs. predictive AI?
- 10.3 3. Q: Can generative and predictive styles work together in harmony?
- 10.4 4. Q: Which industries benefit most from generative AI applications?
- 10.5 5. Q: How crucial is it to consider project goals when choosing between generative or predictive technologies?
Generative AI vs Predictive AI – What's The Difference?
As we delve deeper into this comparative analysis, you'll uncover the essence of generative AI – a masterful creator forging new frontiers by generating data from patterns etched in its digital memory. Step inside a realm where art springs to life, texts compose themselves with eloquence, and imagination dances with pixels. Conversely, predictive AI stands as a stalwart guardian of probabilities, drawing insights from historical footprints to illuminate potential futures. From predicting financial trends to forecasting patient outcomes in healthcare settings, predictive AI casts a visionary gaze across industries far and wide.
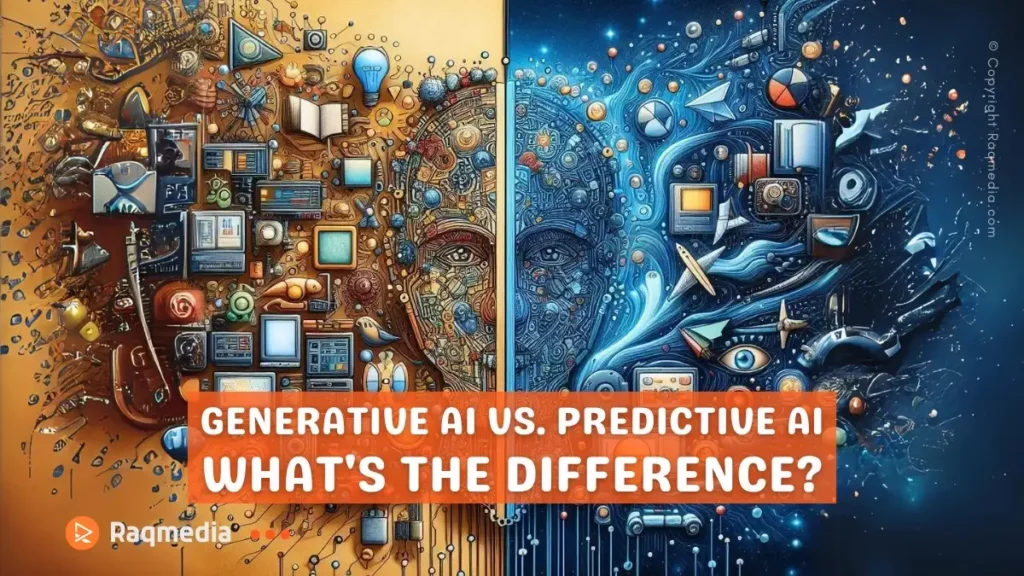
Join us on this enlightening expedition as we navigate through the realms of possibility crafted by generative and predictive AI. Let your curiosity guide you as we unravel the fabric of machine intelligence brick by pixelated brick. It's time to choose your style – will you be captivated by generative ingenuity or spellbound by predictive precision? The answer lies within these pages, waiting for you to seize it with open minds and eager hearts.
Generative AI: Fostering Creativity Through Data Transformation
Generative AI, a fascinating subset of artificial intelligence, stands out for its ability to create new data by discerning and learning patterns from existing datasets. This inventive approach enables machines to produce content autonomously, mimicking human creativity in remarkable ways. A prime example of generative AI's prowess is seen in the realm of art generation. Artists and designers are leveraging generative models to produce unique pieces that push the boundaries of traditional artistic expression. By analyzing vast amounts of artwork data, these algorithms can develop novel styles and compositions that captivate audiences worldwide.
Text Generation Applications
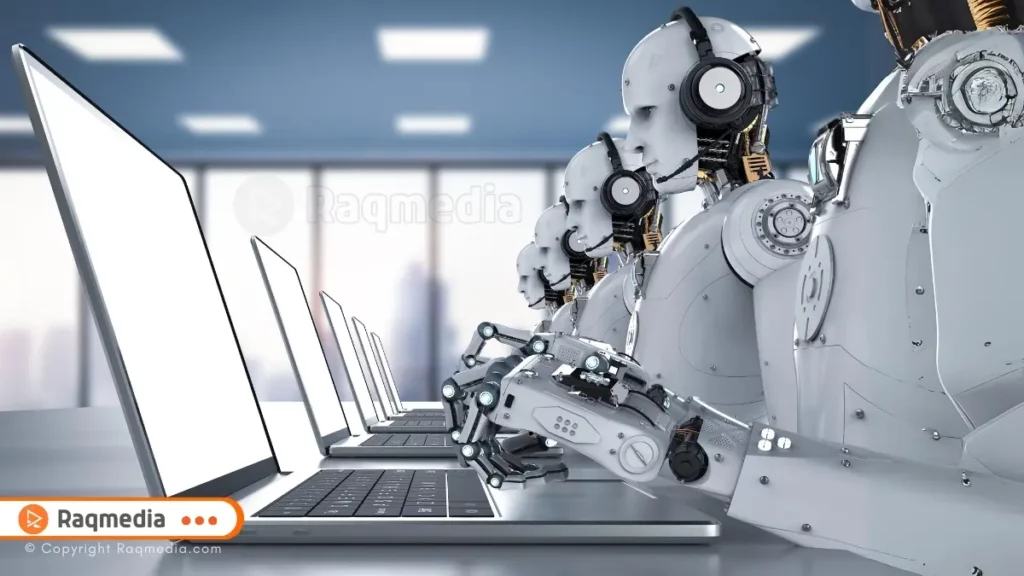
Moreover, generative AI plays a pivotal role in text generation applications, showcasing how it can revolutionize content creation processes. From crafting engaging storytelling narratives to generating realistic dialogues, this technology empowers writers, marketers, and storytellers with tools that optimize efficiency and spark innovation. However, as generative AI continues to evolve rapidly, concerns regarding ethical implications have surfaced prominently.
One such issue revolves around deepfakes—manipulated media content created using generative techniques—that pose significant challenges related to misinformation and trustworthiness. It becomes imperative for developers and users alike to navigate these ethical dilemmas responsibly within the creative realms fueled by generative AI technologies.
In summary, generative AI opens up new frontiers where technology intersects with creativity in unprecedented ways. By harnessing the potential of algorithmic creativity, industries ranging from entertainment to marketing are witnessing transformative advancements that redefine conventional practices. As we delve deeper into the capabilities of generative AI, striking a balance between innovation and ethical considerations will be crucial in shaping a future where artificial intelligence contributes positively to our societal fabric.
Predictive AI: Shaping Tomorrow with Data Insights
Predictive AI, often referred to as machine learning or statistical models, is a powerful tool that transforms historical data into actionable projections for future trends. By analyzing vast amounts of past information, predictive AI enables organizations to make informed decisions and optimize strategies across various industries. For instance, in finance, predictive AI algorithms can forecast stock market movements based on historical trading patterns, empowering investors to anticipate market shifts and manage risks effectively.
In healthcare, predictive AI plays a pivotal role in improving patient outcomes by predicting potential medical conditions based on patients' health records. For example, predictive models can identify individuals at risk of developing chronic diseases like diabetes by analyzing their lifestyle choices and genetic predispositions. This proactive approach allows healthcare providers to offer personalized interventions and preventive measures to mitigate health risks before they escalate.
Despite its remarkable benefits, predictive AI encounters challenges related to biases in the data used for training models. Biased inputs can result in inaccurate predictions and perpetuate inequalities in decision-making processes. To address this issue, data scientists are constantly refining algorithms to minimize biases and enhance the accuracy and fairness of predictive insights. By fostering transparency and ethical considerations in predictive AI development, stakeholders can nurture trust in these technologies and unleash their full potential across diverse domains.
Performance Comparison: Generative vs. Predictive AI
When we delve into the performance aspects of generative versus predictive AI, we uncover distinct strengths and weaknesses that delineate their utility in various contexts. Generative AI exhibits proficiency in creative endeavors like content generation, image synthesis, and even music composition by capturing patterns from existing data to forge something novel. This capability sparks innovation and creativity, as exemplified by Google's DeepDream algorithm, which creates mesmerizing art pieces. However, the Achilles' heel of generative AI lies in its potential for misuse, especially in the realm of deepfakes where fabricated content can blur the lines between reality and falsehood.
On the flip side, predictive AI excels in making informed projections based on historical data trends, offering valuable insights for decision-making across industries like finance and healthcare. For instance, predictive analytics have transformed the healthcare sector by enabling early disease detection through data-driven diagnoses. Despite its prowess in forecasting trends and behaviors with accuracy, predictive AI is susceptible to biases entrenched within historical datasets that could skew outcomes. These biases pose a significant challenge that organizations must navigate to ensure fair and equitable predictions.
To gauge when each type of AI is most suitable for a project, it's essential to align their capabilities with specific objectives or goals. Generative AI thrives in scenarios demanding creativity and originality, such as design processes or artistic productions. On the other hand, predictive AI shines in applications requiring trend analysis, risk assessments, or forecasting future outcomes based on historical patterns – making it indispensable for strategic planning initiatives within businesses or research endeavors. Case studies showcasing successful implementations of both generative and predictive AI offer valuable insights into their practical applications across diverse sectors like entertainment, finance, healthcare, and more.
In essence, understanding the distinctive strengths and weaknesses of generative versus predictive AI empowers stakeholders to make informed decisions regarding which style best suits their project requirements. By leveraging the creative potential of generative AI alongside the analytical precision of predictive models judiciously tailored to specific needs, organizations can harness a powerful blend of innovation and foresight to drive impactful results in an increasingly data-centric world.
User Perspective: Choosing Your Style
When it comes to deciding between leveraging generative or predictive AI for a project, there are several crucial factors to consider. Generative AI can be a great fit when the goal is creative output, such as generating artwork or crafting new pieces of music. On the other hand, predictive AI excels in scenarios where historical data can be used to forecast future trends accurately, like in stock market predictions or disease outbreak modeling.
Industry experts offer valuable insights on their experiences with each AI style. For instance, Sarah Chen, a data scientist at a leading tech firm, mentions that generative AI has allowed her team to explore innovative solutions through creative problem-solving methods. In contrast, Mark Rodriguez, a financial analyst specializing in predictive analytics, emphasizes the power of predictive AI in helping his company make data-backed decisions that drive revenue growth.
To evaluate which style aligns best with an organization's objectives and resources, it's essential to assess factors like project goals, available data sources, and required accuracy levels. Organizations should also consider the ethical implications associated with generative AI applications like deepfakes and weigh them against the potential societal benefits predicted by both styles. Conducting thorough cost-benefit analyses and consulting with domain experts can further guide stakeholders in making informed decisions tailored to their specific needs and priorities.
Future Trends in AI Development
As the realm of artificial intelligence continues to evolve, future trends are shaping the development of both generative and predictive AI technologies. One exciting trend is the increased focus on interpretable AI, especially in predictive models. Researchers and developers are working towards creating algorithms that not only make accurate predictions but also provide transparent explanations for why a particular decision was made. This push for transparency is crucial, particularly in industries like healthcare, where understanding why an AI system recommends a certain treatment can significantly impact patient trust and outcomes.
Moreover, the integration of generative and predictive approaches is gaining traction in various sectors. For instance, in marketing, companies are blending generative AI capabilities to create personalized content based on predictive analytics insights. By harnessing generative models to generate targeted advertisements or product recommendations derived from predictive algorithms analyzing customer behavior data, businesses can enhance customer engagement and drive conversions effectively. This fusion of generative creativity with predictive accuracy offers a powerful toolset for organizations looking to stay ahead in competitive markets.
In healthcare, the future trend leans towards leveraging generative AI for image synthesis combined with predictive analytics for early disease diagnosis. Imagine a scenario where a generative AI model can generate synthetic medical images resembling actual patient scans based on limited data inputs. These synthesized images could then be analyzed by predictive algorithms trained to detect early signs of diseases with high accuracy.
By marrying the creativity of generative AI to produce realistic medical images with the analytical prowess of predictive models spotting anomalies, medical professionals can expedite diagnoses and improve patient outcomes significantly.
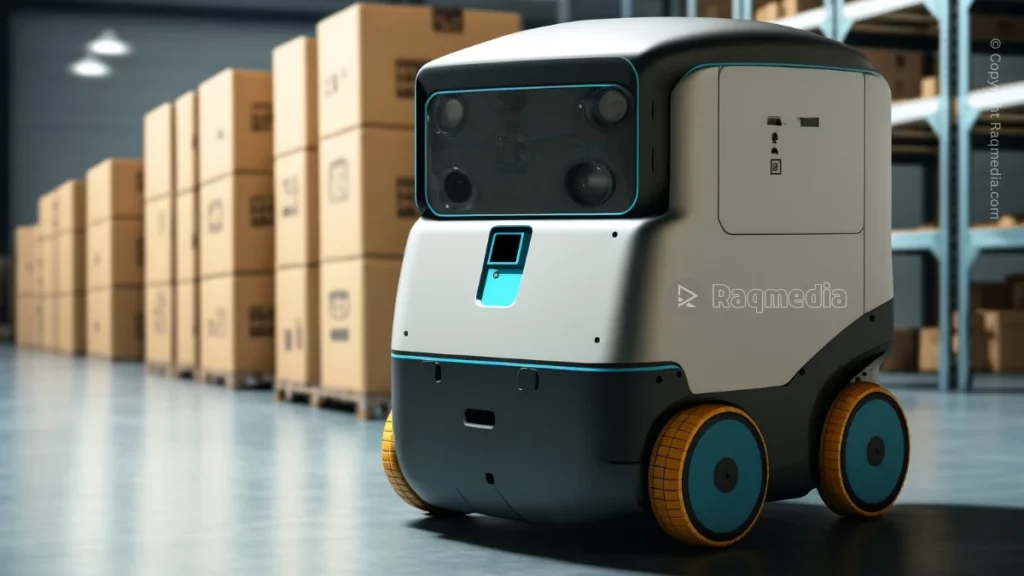
In robotics, integrating generative and predictive approaches opens up possibilities for enhancing robot autonomy and adaptability in dynamic environments. Generative models capable of simulating varied scenarios combined with predictive algorithms predicting potential outcomes empower robots to make informed decisions on-the-go. This fusion enables robots to adjust their actions based on real-time data inputs while creatively generating solutions when faced with unforeseen challenges.
The synergy between generative creativity and predictive foresight paves the way for next-generation smart robots adept at handling complex tasks efficiently in diverse settings – from manufacturing floors to disaster response missions.
Generative AI's Creative Prowess In Question
In the ever-evolving landscape of artificial intelligence, the comparison between generative and predictive AI styles has shed light on their distinct functionalities and applications. Generative AI's creative prowess in generating new data based on existing patterns opens up possibilities in art generation, content creation, and even music composition. On the other hand, predictive AI's ability to forecast outcomes based on historical data finds significant utility in industries like finance for stock market predictions or healthcare for patient outcome projections.
As we conclude this exploration, it becomes evident that understanding both generative and predictive AI is crucial for tech enthusiasts, data scientists, and AI professionals alike to wield the power of these technologies effectively. By delving deeper into the nuances of each approach, practitioners can leverage their unique capabilities to drive innovation and solve complex problems across diverse domains. Whether it's harnessing generative AI to create deepfake detection algorithms or employing predictive models to optimize supply chain management, a comprehensive grasp of both styles can unlock transformative opportunities in AI-driven solutions.
We encourage readers to continue their learning journey by further exploring generative and predictive AI methodologies through hands-on projects, research papers, or industry workshops. By staying abreast of advancements in these fields and experimenting with real-world applications, individuals can gain practical insights into when to apply generative creativity or predictive foresight effectively. Embracing a holistic understanding of these AI styles not only enriches one's professional skill set but also paves the way for groundbreaking innovations that shape the future of technology.
Final Tips
As you navigate the realms of generative and predictive AI, here are three essential tips to consider when deciding on your style:
- Understand Your Data: Whether opting for generative or predictive AI, a thorough understanding of your data is fundamental. For generative AI, training it on high-quality and diverse datasets will enhance its ability to generate accurate and creative outputs. On the other hand, for predictive AI, ensuring your historical data is clean and free from biases is crucial to yield reliable predictions. For instance, in healthcare applications, understanding patient demographics and medical history can significantly impact diagnosis accuracy using predictive models.
- Define Clear Objectives: Before diving into an AI project, clearly define your objectives and desired outcomes. Generative AI might be more suitable when creativity and innovation are key goals, such as in designing personalized marketing campaigns based on customer preferences. Conversely, predictive AI excels in scenarios where making informed decisions based on historical patterns is critical, like predicting sales trends for optimizing inventory management in retail settings.
- Continuous Learning and Evaluation: The field of artificial intelligence evolves rapidly with new advancements and techniques emerging constantly. To stay ahead of the curve, fostering a culture of continuous learning within your team is vital. Regularly evaluating the performance of your AI models against predefined metrics ensures that you adapt to changing requirements effectively. By embracing ongoing education and assessment practices, you can refine your approach with either generative or predictive AI to achieve optimal results.
By incorporating these tips into your decision-making process when selecting between generative and predictive AI styles, you can harness the full potential of artificial intelligence to drive innovation and success in your projects while staying abreast of industry developments.
Choosing Your AI Style
In the dynamic realm of artificial intelligence, the juxtaposition between generative and predictive AI opens a world of possibilities for tech enthusiasts, AI professionals, data scientists, and students alike. Generative AI sparks creativity by reimagining what is possible through fresh outputs, while predictive AI navigates with precision through actionable insights drawn from historical data. Each style presents its strengths and limitations, nudging decision-makers to carefully consider their project goals, ethical implications, and resource constraints before taking the plunge into one camp or straddling both for optimized outcomes.
As you embark on your AI journey, remember that selecting the right style—generative or predictive—can be pivotal in unlocking the full potential of artificial intelligence for your endeavors. Keep yourself informed about emerging trends in AI development to stay ahead of the curve and pivot effectively in an ever-evolving technological landscape. Whether crafting art with a brush woven from neural networks' strokes or predicting financial markets with astonishing accuracy, your choice between these contrasting styles can dictate not just outcomes but also shape industries and redefine innovation boundaries.
FAQs
1. Q: What are some key differences between generative and predictive AI?
A: Generative AI creates new data patterns based on existing information, fostering creativity. Predictive AI focuses on making projections using historical data to guide decision-making.
2. Q: How do ethical concerns come into play when discussing generative vs. predictive AI?
A: Generative AI may raise issues like deepfake technology, whereas predictive models can exhibit biases based on historical data, leading to unfair outcomes.
3. Q: Can generative and predictive styles work together in harmony?
A: Yes! Combining generative's creative outputs with predictive's analytical prowess can offer comprehensive solutions bridging innovation and practicality.
4. Q: Which industries benefit most from generative AI applications?
A: Industries like design, storytelling (text generation), and advertising leverage generative AI for innovative content creation tools.
5. Q: How crucial is it to consider project goals when choosing between generative or predictive technologies?
A: Understanding your objectives helps determine whether you need imaginative content creation (generative) or accurate projections (predictive) tailored to your industry requirements.
🤖 Get ready for an ultimate showdown: Generative AI vs Predictive AI! Understand the differences and choose your side wisely. 🚀📧🔗 Read more at:
https://www.raqmedia.com/generative-ai-vs-predictive-ai/
👍 Don’t miss out! Follow and like Raqmedia for more valuable insights!
#raqmedia